
Petia Radeva
Head of Medical Imaging Lab, Computer Vision Center
Head of Consolidated Research Group “Computer Vision and Machine Learning at the University of Barcelona”, University of Barcelona, Spain
Medical Imaging has represented a real revolution in healthcare over the past 30 years. Today, it is difficult to think about having some important disease or clinical procedure without using some kind of medical images (radiography, computed tomography, magnetic resonance, ultrasound images, etc.). Images are used for prevention, diagnosis, therapy, intervention, and follow-up. Hence, the New England Journal of Medicine considered imaging as one of the top medical developments of the past 1000 years.
Medical imaging is defined as the technique and process of creating visual representations of the inner body for clinical analysis and medical intervention. It reveals internal structures hidden by the skin and bones in order to diagnose and treat disease. Medical imaging is generally classified as either Anatomical or Functional. Most medical images use so-called “invisible light”. Usually, images are obtained using special signals or waves (X-ray radiography, MRI, CT, UC, endoscopy, elastography, tactile imaging, thermography, PET, SPECT) to interact or explore anatomical or functional properties of the tissue.
Relatively, recently, standard digital video or still pictures have been used like in dermatology or wireless endoscopy. Thus, a natural question arises: can standard conventional images and videos be useful and important for health applications? What could be the new applications of Computer Vision for healthcare? Examples of such applications could be: using a Kinect video to detect persons in agitation in Emergency Care Units, using video to analyze the gait of Parkinson patients and follow-up the progress of the disease, using video to observe behavior of TDAH children or using a wearable camera to analyze the behavior and context of individuals to predict migraine or epilepsy attack.
The Medical Imaging Lab (MILab) of Computer Vision Center (www.cvc.uab.es) that is also part of the Consolidated Research Group “Computer Vision and Machine Learning at the University of Barcelona” (CVUB) – Spain, is exploring new horizons for the use of Computer Vision and Deep Learning applied to healthcare as a complementary to medical imaging.
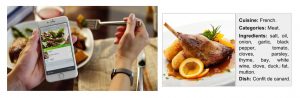
Monitoring and analysis of nutritional behavior of people to prevent chronic diseases. Diet and nutritional habits are one of the 4 main risk factors for many diseases like diabetes, obesity, cardiovascular diseases, and also are related to cancer and neurodegenerative diseases (Alzheimer). However, today we do not have easy and objective tools for monitoring food intake, unlike to the numerous monitoring tools for physical activities (e.g. Fitbit). In order to follow nutritional behavior, individuals should annotate each meal in a food diary, which makes the process both tedious and time-consuming. Having an app on a smartphone can greatly facilitate food intake recording. However, food recognition must be automatic in order to be fast and easy to manage. CVUB has developed strong and robust food recognition algorithms that are able to recognize food group (pasta, meat, etc.), food class (e.g. pizza, codfish with lemon), food ingredients or even food cuisine with a precision higher than 90%. In this way, physicians, behavioral psychologists, and nutritionists can have continuous and objective information about an individual’s diet and provide much more effective intervention.

Pictures captured by a smartphone show what people eat. But they miss information of the context of eating (where, when, with whom, how) that recently has been discovered to have a great role in the nutritional behavior of individuals. Using a wearable life-logging camera can capture images of the context and provide this information. CVUB has developed strong deep learning algorithms to extract and discriminate the food environment, social environment, as well as contextual events before and after food intake in order to provide new evidence and data for improved behavioral intervention. The real effect of such intervention can be explored through medical imaging like functional magnetic resonance images taking into account that metabolic diseases like obesity are characterized by different activation patterns of brain regions. We are still at the beginning of exploring how medical and conventional images can be combined for a more holistic monitoring of individuals, efficient intervention of individual behavior and helping healthcare to move from diagnosis and therapy to prevention of chronic diseases.